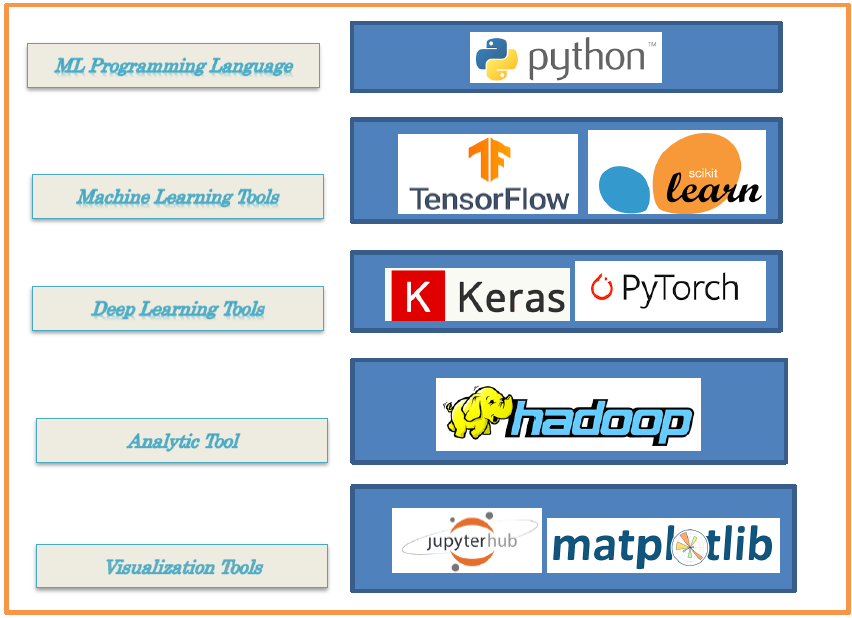
NCS AI & ML Platform is an Deep Learning Platform that helps you to accelerate your journey to cognitive computing by bringing together a collection of the most popular open source frameworks for deep learning, along with supporting software and libraries in a single installable package.
Artificial Intelligence (AI): Artificial refers to something which is made by human or non-natural thing and Intelligence means βthe ability to acquire and apply knowledge and skills.β There is a misconception that Artificial Intelligence is a system, AI is not a system .AI is implemented inside the system. To make things simple, Artificial Intelligence is the replication of human intelligence in computers.
Machine Learning (ML): means the application of any computer-enabled algorithm that can be applied on a data set to find a pattern in the data. This encompasses basically all types of data science algorithms, supervised, unsupervised, segmentation, classification, or regression.
Applications of Machine Learning:
- Handwriting Recognition – Convert written letters into digital letters
- Language Translation – Translate spoken and written languages (eg. Google Translate)
- Speech Recognition – Convert voice snippets to text (e.g. Siri, Alexa)
- Image Classification – Label images with appropriate categories (e.g. Google Photos)
- Autonomous Driving – Enable cars to drive (e.g. Google Car)
The AI & Deep Learning suite of framework software will be embedded in this project across any/all relevant GPU-based compute nodes. These applications are extremely relevant to Deep Learning today as the leading institutions are transforming their education, research and industry collaboration activities for these new domains. The hardware and software platform for Deep/Machine Learning brings unique advantages to the table.